Leverage Big Data and Machine Learning
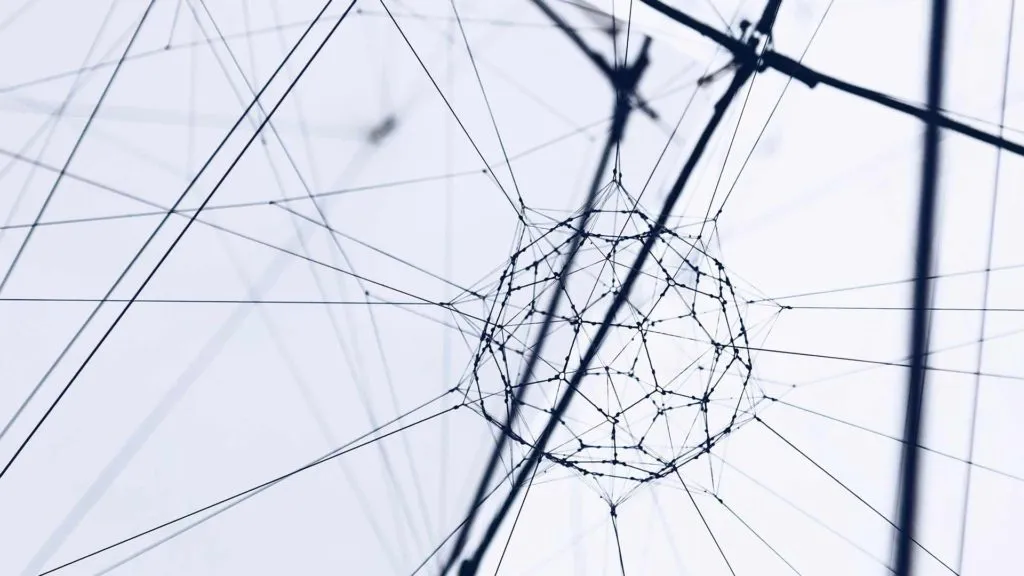
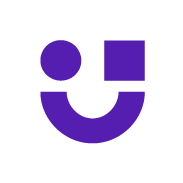
Digital transformation is disrupting the basic approach that companies have to business and their corporate strategy.
This change in mentality requires businesses to reconsider and redesign processes to comply with the new rules of the digital era. Innovation and speed are two important factors, but the main force that is shaping digital disruption is customer experience.
Digitization allows firms to tailor processes, roles and operations to meet their customer needs.
Once the process of transformation becomes formalized and standardized, companies often employ a chief digital officer to implement and structure changes. As son as these changes become systematic and institutionalized it is time for companies to implement the new strategy and actually move towards the new goals.
Since customer experience is the main driver of a digital strategy, marketing, being closest to customer expectations, becomes the unit responsible for leading digital transformation within corporations.
Marketing assumes a pivotal role in change management and a modern SaaS marketing strategy must be based on data-driven decisions that create the perfect substrate for communication, lead generation, product management, sales, and customer development.
Table of Contents
Customer Intimacy
The first important element of a modern marketing strategy that supports digital transformation is customer intimacy: the ability to interact with each single client at a personal level.
The classic marketing principle of segmentation is now completely outdated. Sure, there might be some common aspects that can be analyzed and used to address different portions of the population, but the basic concept of high-level data aggregation does not apply to digital strategy.
In the past, we didn’t have the means to collect specific data and we were forced to group prospects on the basis of simple demographics such as age, gender, income, geographic data and so on.
This classic segmentation can still be useful to identify promotional channels and values to design a solid communication strategy. But digital technology offers companies the opportunity to access granular data that allows us to address each single prospect individually.
The more hyper-personalized and individual the approach is, the more sophisticated our marketing strategy becomes.
Artificial Intelligence and Machine Learning for Marketers
Changes in a modern marketing approach are driven by big data, analytics, and customer intimacy.
The tools that enable the process are therefore connected to all those technologies that enable data mining, visualization, and analysis.
But the whole digital transformation for marketing doesn’t stop there.
Essential instruments of a digital strategy include automation, predictive analytics and modeling, Artificial Intelligence (AI), and Machine Learning (ML).
All these elements need to be carefully orchestrated to create a pleasant customer journey and a great customer experience while addressing customers individually based on their specific needs.
The aim is creating clear communication that leads prospects to become loyal customers without any friction.
New digital technology affects the way companies present their brand, create marketing campaigns and all decision-making processes connected to strategy and problem-solving.
Let’s quickly look at the main elements of the digital strategy to see what role they play in the new marketing approach.
Modernize your Saas Marketing Strategy in Steps
The process of digitization of an Saas marketing strategy goes through different phases and these are the main stages you’ll be going through:
- Define objectives and start with a hypothesis
- Decide what processes can be streamlined to test hypothesis
- Define the data you need
- Implement technology that allows you to collect insights at each stage of the customer journey
- Collect data from all different sources and work on quality
- Centralize data and allow access to every unit
- Organize and structure data
- Create metrics and KPIs
- Set initial goals and draw up strategy
- Implement AI and ML to automate, tailor and speed up processes + collect and analyze data
- Focus on customer experience
- Monitor results and adjust strategy
The implementation of Big Data, ML and AI occurs at different stages of the digital strategy: some tool are used during the preparation while other tools can be used to define objectives, implement the tactics within the strategy and monitor results.
Big Data and SaaS Marketing Strategy
Everything starts with data. Without data there can’t be any transformation.
A data-driven strategy leads to risk optimization and creates the substrate for a modern marketing approach.
Big data for marketing doesn’t simply refer to gathering a huge amount of random information about existing or potential customers. In order to make a dent in the whole marketing strategy, data needs to have specific characteristics and the approach to data needs to be systematic.
Data quality
The first problem that SaaS companies often incur is data quality. This primarily derives from the size of the original dataset which is collected and analyzed.
Large B2C companies deal with millions of customers who often buy their products on a regular basis. Consumer goods are basic commodities acquired by single individuals for which purchasing behaviors and sales cycles are easy to visualize and analyze.
SaaS companies deal with entire entities and the number of existing customers often doesn’t represent a significant sample for modelling predictive analysis.
Samsung can collect millions of data points in a single quarter whereas B2B companies rely on much smaller poll information collected over long cycles and are often forced to access data by analyzing competition.
Talking about quality, then, data can become fragmented and duplicate information can lead to wrong assumptions.
Similar problems occur when different departments manage and access different data. How to avoid that?
Avoid tunnel vision and enforce cooperation and integration between different units.
In order to set the stage for a solid digital strategy, sales, marketing, product management, engineering, customer development and customer support need to cooperate and share as much information as possible.
Sales reps and product managers should exchange information with marketing and all the data that separate units collect must be centralized and made consistent.
Duplication and fragmentation spoils the data and by not forcing different department to sit at the same table, companies often miss on the opportunity to create a homogeneous and smooth customer experience.
Different stages of the customer journey provide precious insights that need to be collected and used strategically to improve product, promotion, and sales approach.
Companies often deploy different applications at different stages, therefore, it is vital to connect data applications and allow them to exchange data. A key element of data centralization and quality is the integration of different software solutions used by different units to gather information.
Humans still play a big role in terms of quality when data input needs to be done manually.
A sales rep that doesn’t enter all the information they collect when talking to a potential client or a product manager that interprets customer survey data in the wrong way can be factors that deteriorate the overall quality.
Companies need to put in place a systematic approach to data collection that needs to be enforced at each level while units must regularly exchange information and cleanse business intelligence data.
Data Structure
A “clean” dataset is not the only element that allows companies to utilize the information they collect.
The next point is how you organize, structure, visualize and analyze the information they gather.
It is important to know how to structure data for analysis and reporting but also be aware of how this information can be centralized and accessed by different units.
After creating a proper infrastructure for data collection and analysis, companies need to find a way to structure it in a way that makes sense for their business model.
The more granular the data, the stronger its impact on customer intimacy, but information still needs to be aggregated to be reported and implemented in a digital strategy.
Data structure plays an essential role in future decision-making processes and can quickly lead to wrong assumptions if not properly set up.
Big Data Applications for SaaS Marketing
According to Gartner, big data is not solely defined by Volume but also by its variety and velocity. Variety refers to the different media we use to represent data and that go beyond simple figures. Velocity is connected to the speed at which data is collected, analyzed and implemented. Additional characteristics of data include Veracity (clear and unambiguous information), Value (how relevant and up to date the information is) and Variability (to provide an unbiased perspective).
SaaS firms, besides organizing and collecting business intelligent data internally, can also implement applications that allow companies to collect and structure publicly available data. Some of these data can simply be a byproduct of online activities which are collected electronically, other information can be systematically gathered and reported by specific organizations.
Access to data can be free but we’re currently seeing the rise of services whose business model is based on selling third party data or services that rely on it.
The data SaaS companies collect need to be relevant and recent. The more up to date the data is the more efficiently it can be implemented.
Large corporations can access data collected through loyalty programs and cross-promotional activities. Smaller businesses can acquire data through customer surveys, online tracking and by analyzing competition, but strategic partnerships within the same ecosystem can also be a golden opportunity for SMBs to broaden their perspective on potential customers.
Many applications that SaaS companies use to communicate and support users also provide analytics and precious information regarding the behavior of existing customers.
Main sources of data are freely accessible and include tools like Google or Social Media analytics.
Customer profiling solutions for B2B companies allow to collect information through social profiles of their customers, learn more about the specific language they use, the approach they prefer and discover behaviors which occur outside of their platform. Using this information in marketing and sales communication dramatically improves efficacy and activation.
After acquisition, data needs to be organized and visualized. There are several products that provide data analysis through infrastructure virtualization (cloud computing) and specific algorithms that allow to reveal patterns that might not be immediately obvious.
Additionally, companies can look into specific solutions that are specifically designed to analyze customer experience, gather customer comments and opinions from different sources (blogs, forums, social media), analyze the general sentiment and opinion of their customers and prospects (voice of the customer applications – VoC) for reputation management.
All this information can be used to define the customer persona, shape marketing communication, branding, and for adapting the product to customer expectations. Social listening tools and customer voice analytics are essential elements of a strategic marketing planning approach that puts customers at the center of the picture.
Brand reputation tools and analytic algorithms help businesses re-think their marketing approach and empower users to impact the development of the company.
In essence, a modern brand has to be malleable enough to be able to adapt to the specific requirements of the target group it addresses while still maintaining alignment with its core values and mission statement.
Data Lakes for SaaS Marketing Integration
Creating centralized, flexible repositories for structured or unstructured data is a vital component of a digital strategy.
73% of B2B companies are currently planning on investing a large amount of their budget in creating marketing data lakes. The market for data will increase its volume by 50% over the course of the year but the real challenge for companies is then dealing with all the information they collect.
Source
Again, according to Gartner, most of the businesses feel that they still have no proper infrastructure in place to analyze and implement this data in their marketing strategy and that’s partially why not all companies feel the necessity to invest in big data.
Key to the future success of SaaS businesses is data governance and management.
The main scope of a functional data lake structure is departing from disconnected data silos and rigid data warehouse structures to collect data that marketing automation, CRM, and sales applications generate through reporting and create a comprehensive and cohesive overview within an enterprise data hub.
This infrastructure allows SaaS companies to make informed decisions based on data points collected at different stages of the customer journey and match metrics and performance analytics with the new digital strategy.
Having all the data in a centralized location, easily accessible to different units provides a 360 degree picture of the entire customer experience.
Raw data can be then structured for specific use cases to carry out data management transformations, designing analytics, and leading innovation.
Data lakes are valuable for quick cloud computations and batch processing after addressing all the quality and structure issues mentioned above and allow a rapid ingestion of raw data that meets all the requirements connected to variety, velocity, volume, variability, veracity and value.
Whereas B2C companies deal with single users, SaaS companies deal with account based data and this has a deep impact on the whole data management structure that needs to be put in place.
Analytics are based on long cycles and need to relate to specific metrics such as MRR, churn and growth. The challenge here is connected to the fact that a similar ecosystem is hostile to predictive analytics.
There’s no one-size fits all solution to the problem, but Implementing proper solutions for data governance while maintaining significance and relevance is going to play a major role in the successful implementation of a marketing strategy.
Utilizing Big Data for Drafting a Powerful Marketing Strategy
Account analytics, operational and innovation metrics, together with predictive data influence different aspects of product marketing and promotional strategy.
This, in turn, affects all the elements of sales and marketing, from lead generation to pricing and sales pitches. Big data algorithms can affect decision processes that involve channels and communication while impacting sales operations and marketing solutions,
Big Data affects the quality of the leads generated by populating and enriching prospecting lists with accurate information.
Other areas affected are conversion rate optimization (CRO) based on behavioral analytics, prospect engagement and customer lifetime value (CLTV), customer acquisition costs (CAC), churn and other specific metrics directly connected to a SaaS business model.
B2C companies utilize big data for managing price discrimination for inelastic commodities and pricing decisions are now moving away from standard experimentation and assumptions to reach capillary precision in terms of willingness to pay at customer-product level.
The same may apply for subscription based business models and service level agreements at an account base level if the pricing strategy of the SaaS company can be optimized and adapted.
Customer segmentation and first-party data lead to a more individual approach with individual accounts that lead to a winning personalized customer experience.
After all, according to McKinsey, the majority of customers base their subscription decisions on the overall experience rather than on the validity of the product.
Being able to create flexible, individual customer journeys is made possible by investing time and resources in analyzing and comprehending customer expectations individually.
According to a Forrester study, marketers are now using data mining to improve responsiveness and create account-based marketing plans that are relationship-driven.
In a sense, big data and artificial intelligence are actually making marketing more human, in that they create the basis for a personal interaction with each single customer.
This phenomenon is a paradoxical element of digital transformation which is partially linked to the abundance of providers and resources.
In a market in which creating a positive online footprint is progressively more accessible to anybody, it becomes quite difficult for prospects to judge businesses and products. In turn, it becomes always more difficult for businesses to gain trust and convey their personality through the chaotic noise created by online communication.
Knowing what information is actually relevant for customers is necessary in order to communicate advantages and differentiate from competition. With a limited window of attention, companies need to condense the most salient characteristics of their solution and present the most relevant facts in an aptly manner in all top of the funnel activities.
Collecting user data during trials allows companies to optimize their product and user experience in order to reduce churn.
This optimization leads to faster customer acquisition and increased customer lifetime value.
The modern marketing stack includes a series of applications that collect customer experience and engagement data at different stages of the journey and convert this data into actionable tactics within sectors like:
- Email Marketing (to optimize reach, copy, frequency)
- Content Marketing (content, keywords, distribution channels, virality)
- Personalization and Loyalty Management
- Brand and Reputation
- Funnel structure and Lead-to-Revenue Management (lead generation, funnel activities, CRO)
- Cross-promotions and partnerships
- Multi-channel campaigns
- Retargeting
- Social Media Marketing (channels, content curation, communication strategy)
- User Experience
- Search Engine Optimization
- Influencer Marketing
- Performance Marketing
Given the complexity of information necessary to optimize every sector, each of these elements will be the subject of future articles.
The main takeaway is the fact that attribution, data collection, visualization, analysis, and organization allows marketers and sales reps to improve customer-relationship, gain trust, and increase loyalty.
The same data allows product managers to optimize product cycles, innovation and releases that match customer expectations and lead to higher customer retention, the key factor that differentiates market leaders from contenders.
Big Data is therefore not only used to optimize KPIs and define key drivers of business operations but also to create predictive algorithms that transform the overall approach to product and go-to-market strategies.
Customer Value Data (CVA) provide insights into sales cycles and create a consistent omnichannel strategy through awareness, activation, delivery, experience, support and retention.
Each step of the supply-chain is reinforced with value adding activities triggered by the expectations of existing and prospect customers. Lean operations and high level of responsiveness are now the focus of a solid SaaS marketing strategy.
Preventive and reactive customer support solutions must be implemented to guarantee a smooth experience throughout the entire customer journey.
Strategic experimentation together with a selection the right tools for data orchestration and governance result in the creation of realistic enriched proxies that can be used for lead generation and adjustments to the overall marketing strategy.
But data is not only useful when it comes to lead generation!
Hotjar recently published a very comprehensive guide on how to improve customer experience in which they provide a very extensive list of trends, and examples. By looking at the survey, it is clear that data and customer intimacy are the keys to creating a customer-centric strategy.
Customer experience leaders prioritize a great CX over higher margins in order to create and retain more loyal customers.
Data collection and direct customer feedback provide the basis for a frictionless customer journey that starts with discovery and awareness through marketing activities and leads to advocacy.
Automation, Artificial Intelligence, and Machine Learning and SaaS Marketing
Since leveraging data seems to be the only way for marketers to surpass competition and the most critical aspect of a solid digital strategy, automation, artificial intelligence, and machine learning become necessary to manage complex tasks and tenon information collected in different environments.
Similar technology was once only available to a handful of highly-skilled technicians. Over the course of the past couple of years, we’ve seen a large number of services in this sector flourishing and offering ubiquitous access to advanced resources also for non adepts.
Product recommendations, semantic searches, contextual analytics are implemented in consumer platforms and mobile applications we use every day.
We are all pretty familiar with everyday AI applications that predict what word we’re going to use next in a message with contextual analysis or that predict what product we may like based on design features.
For SaaS companies, similar tools are precious for lead generation, detecting buying signals, and for boosting engagement and retention.
Artificial Intelligence allows to make sense of critical behavioral and contextual data to create better brand stories and to create adaptive platforms, predictive interaction and personalized user experience.
Artificial Intelligence is also used to automate initial communication and customer support while Machine Learning (the ability to learn without being programmed) allows systems to autonomously learn from data and optimize problem-solving processes.
The applications are infinite, from planning and automating a content curation and social media strategy for creating a fan base to campaign optimization.
The important aspect that needs to be highlighted is that data and artificial intelligence can provide guidelines but the real storytelling still needs to be crafted by humans.
Probably, in the near future, machines will be able to replace marketers in virtually every aspect of the brand storytelling and customer journey support. But, so far, automation can streamline operations and show the direction while the real interaction and communication with the customer still needs to remain a human domain.
Interactive content and direct feedback can move the conversation to a personal level and ML can provide the basis for a one-to-one relationship by providing insights on tone and sentiment together with facts and data.
Artificial intelligence and machine learning can be implemented in both product and brand management by allowing marketers and product managers to make sense and utilize data for their strategy.
Source
In the B2C world, companies use AI to personalize advertising based on sentiment or to create interactive marketing campaigns that involve each single viewer in the story. Manufacturers even implement AI to design the product itself.
There’s a plethora of creative solutions that companies implement in order to personalize their story and make people feel part of their brand.
SaaS companies can still use similar creative solutions to create ad hoc marketing campaigns, and tap into the opportunities created by algorithm-driven UI and UX design but things are a bit more complicated in terms of product customization.
When it comes to onboarding new customers, SaaS companies can use smart solutions that allow them to simplify the process for example by offering interactive content based on user segmentation or simply facilitating signups by pre-filling information that can be collected automatically (as it happens with flight booking services for example).
When potential customers interact with the product, SaaS companies have plenty of options to automate and personalize support and the whole experience.
AI and ML can be used for far more than simply creating chatbots or predicting what movie you’ll probably like.
Artificial Intelligence allows marketers and product manager to ditch high-level data aggregation and retrieve actionable advice from granular data.
Direct survey data, indirect customer voice (social listening) and inferred voice analysis (through behavioral observations and modeling) allow product managers to redesign their solution through democratization and AI helps marketers tailor communication and present the brand.
By introducing smart applications in their marketing stack, marketers are now able to access and categorize more data than ever before in an environment, such as the SaaS market, which is truly global.
Making sense of this data and using it for apt marketing decisions is what separates the top SaaS businesses from the others. Success is tightly bound to data-driven decisions and lean approach to strategy.
It all boils down to creating a thorough customer journey map and use smart data to shape and enhance customer experience at each stage.
AI, automation, and ML should become instruments that take away work from marketers so that we can all concentrate on strategic decisions and on building relationships with prospects, customers, partners and influencers. These tools are aids and not a replacement for a strong marketing team.
The Challenges that Marketers, Engineers, and Product Managers Face in the Digital Era
Key elements of a digital strategy require SaaS businesses to provide on-demand, self-service solutions while providing a high level of support for each single user.
Effortless integration of different services and compliance with basic standards are other important factors.
Simply put, customers demand immediate access from everywhere at any time and maximum freedom in terms of customization and integration.
Marketers, product managers and engineers need to work together in order to meet these new requirements.
Market leaders are shifting their focus from the short-term goals to the long-term value of each single customer. The focus of a modern SaaS marketing strategy needs to be customer experience and maximizing the value of its product and content communication.
The optimization of the digital strategy through big data, artificial intelligence, and machine learning should support companies that want to move from from single the point-of-sale transaction to a long-lasting relationship with their customers that generates loyalty and referrals.
Marketing is the key driver of digital transformation and tech-savvy marketers who are strongly relationship-focused and have a solid, long-term strategic approach to customer experience are the CMOs that are going to carry the leadership and oversee digital disruption in modern SaaS companies.
Ready for the journey?
New to Userlane? Take a step in your software adoption journey and join the pool of our happy customers.
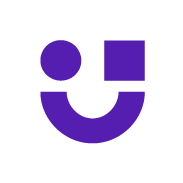